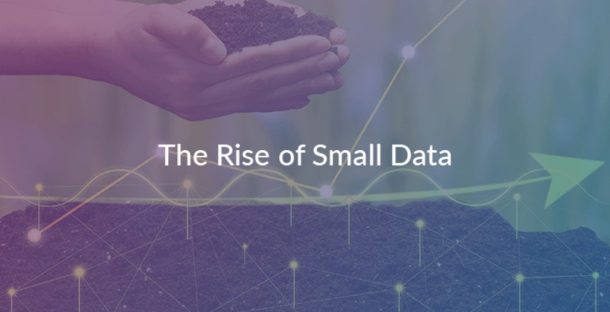
NAA Member News: Senseye – the secret to avoiding data overwhelm
Alexander Hill, Chief Global Strategist at Senseye, looks at why small data is crucial when it comes to expediting asset management and supercharging Industry 4.0 across industrial plants.
Once upon a time, a product’s unique selling points (USPs), patents, or the customer experience (CX) typically represented the intellectual property (IP) of a business.
Fast forward to today, the most valuable commodity in any business is, without doubt, data. When you consider that we produce an estimated 2.2 quintillion bytes of data every single day, it’s no wonder.
And in fact, The Economist claims that data has overtaken oil as the world’s most valuable commodity. It therefore stands to reason that in order to control the direction of a business, organisations need control over the data they process. Big data is clearly one of the biggest buzzwords of the last five years. However, on its own, it only seeks to paint a picture of data overwhelm.
Finding the needle in a haystack
One area in which the right data strategy can easily deliver value is asset intelligence, as it delivers immediate reductions in downtime and labour. But getting it right is a challenge. Many organisations have learned this to their detriment and in their quest to leverage big data, have opted for models which generate an inordinate amount of information.
In a typical automotive environment for example, a robot can generate up to 50GB of data per day. When you consider that a body shop might use 2,000 robots, in addition to transformers, conveyors and welders, all of which make up a plant, it’s easy to become completely overwhelmed. 100,000GB data per day as a minimum is incredibly difficult to store, let alone analyse, and is akin to looking for a needle in a haystack that just keeps getting bigger and bigger – not to mention the fact the needle keeps getting buried deeper and deeper in the process. Meaningful insights are therefore challenging to extract via this kind of approach. And while the maturity of Machine Learning (ML) and Artificial Intelligence (AI) hold immense potential to add value, when faced with data on this scale, they consume a great deal more energy and require substantial data scientist expertise.
The rise of Small Data
In our experience the smartest factories are now adopting a “small data” model, distilling a pre-defined set of data points from which to derive meaningful insights from the swathes of irrelevant data. With the focus very firmly on value over volume, this approach involves setting out precisely the types of data needed to ascertain and analyse a particular pattern or outcome.
Condition monitoring typically requires just a few metrics per machine to detect faults and identify patterns, and for a relatively small amount of input and data collection, significant value can be sought.
Furthermore, data doesn’t necessarily need to be collected in real-time, but at pre-set intervals pertinent to the function of the machine. For some this could be hourly, for others daily, but through switching off the constant data flow, data volumes are substantially reduced. Rather than being drowned out by the sheer noise of the data, a focus on what is relevant, undertaken in a considered pragmatic way, with an experienced steer to ensure the right focus is inordinately more effective.
In a recent example, applying this model against an objective focused on condition monitoring and capability, a targeted 200kb of data from 2,000 robots was generated, with the process of distilling analysis and conclusions quicker and more focused than a big data approach.
The rise of the asset passport
Technology is of course only half the story and through using a platform such as Senseye’s PdM Omniverse, asset experience is built in to work through the how, when and why of which metrics to apply.
Through leveraging this experience and expertise, dedicated ‘asset passports’ can be created, which identify what to look for in a machine, including significant attributes, speed, and the impact of modifications.
This can then scale to additional equipment or expand to look at certain aspects of performance in more detail. The ROI associated with such a targeted “small data” approach is vast, as evidenced in Senseye’s ROILock®.
Senseye customers typically find that Senseye PdM reduced unplanned downtime by up to 50%, which is more than enough to recoup the cost of deployment within weeks or months, rather than years. In addition, users can experience a productivity boost of 55% and an increase in maintenance accuracy of 85%.
But perhaps most importantly, this model delivers the potential to prove value to the business, build future business cases, and educate on how data can be leveraged to reduce downtime, boost performance and enhance safety in the long term. Small data, distilled at the right time, in the right way, keeps the data aspect of asset performance simple and scalable, and while delivering value in the short term, provides a robust and flexible foundation for the future.